How We Learn (Part 1)
How to get really good at anything you want (except juggling we don't cover juggling)
I’ve divided this essay into two parts, because it got very long, and there were two halves to the book. This is an essay about How We Learn, by Stanislas Dehaene. I’ve actually started with the second half of the book, which discussed his four pillars of how to learn. If you want to improve the way in which you learn, this post will cover many of the critical ideas. In the next post, we’ll look at some of the other aspects of learning that he discusses, like the ability to manipulate memories and the neuronal recycling thesis.
According to Stanislas Dehaene, learning should be defined as the construction of a mental model about the world. You probably have thousands of mental models, that range from how you fry an egg to how electrons work. Your mental model for frying an egg is probably pretty good. Unless you are a physicist, your understanding of how electrons work is probably pretty bad, but you were probably taught about electrons at school, and so you probably have a mental model of how they work.
Mental models are constructed, hodgepodge, from the myriad things we learn over time. The fundamentals of such schema remain in line with ideas about active inference - you create theories about how the brain works, and you test these, and refine your theories over time. This happens in all aspects of thought from perception to abstract knowledge.
There are people who write a lot about mental models, and if you are familiar with these, the term can be misleading. If you’re not familiar with them, please don’t read about them now! It’ll get confusing and I’ll have to start again.
Those mental models, the corporate branch of the mental model world, so to speak, are best thought of as derivative constructs from the more fundamental mental models Dehaene is thinking about. Dehaene’s mental models are not specialised tools for thought, to be wheeled out when making important decisions. Instead, Dehaene’s mental models are a way of analogising how your brain perceives the world in a way that is coherent with how the brain works. Hopefully.
For instance, you likely have a mental model that you can’t put your hand through a table. This is not a useful mental model for making difficult decisions in a changing corporate environment, but it is a useful mental model for when you want to eat lunch.
How are such schema constructed? Well, they are partly innate. Babies seem to have an innate sense that lunch can’t go through tables. But babies spend a lot of time throwing that same lunch everywhere to see where it can go, which we could call ‘mental model testing’.
This touches on the first big myth that Dehaene sets out to tackle: the idea that the mind is a tabula rasa, or a blank slate. This comes in large part from John Locke, and was developed by other thinkers like Jacques Rousseau. The blank slate argument is simple. Locke proposed that our minds are blank slates that are moulded by our experiences. Anything becomes possible with the right set of experiences.
This is not true.
Experiences are important for our brain, but basically shape the last few millimetres of cortical wiring. You can’t rewire your auditory cortex into a visual cortex. Accordingly, we begin our voyage into learning with someone who rewired a ferret’s auditory cortex into a visual cortex.
Auditory signals normally travel from the cochlear to the brain stem, via the vestibulocochlear nerve and the medial geniculate nucleus (MGN). Mriganka Sur severed these connections in some ferrets. The ferrets became deaf, and could no longer listen to their favourite ferret artists like Chris Pine Martin and Polecat Stevens.
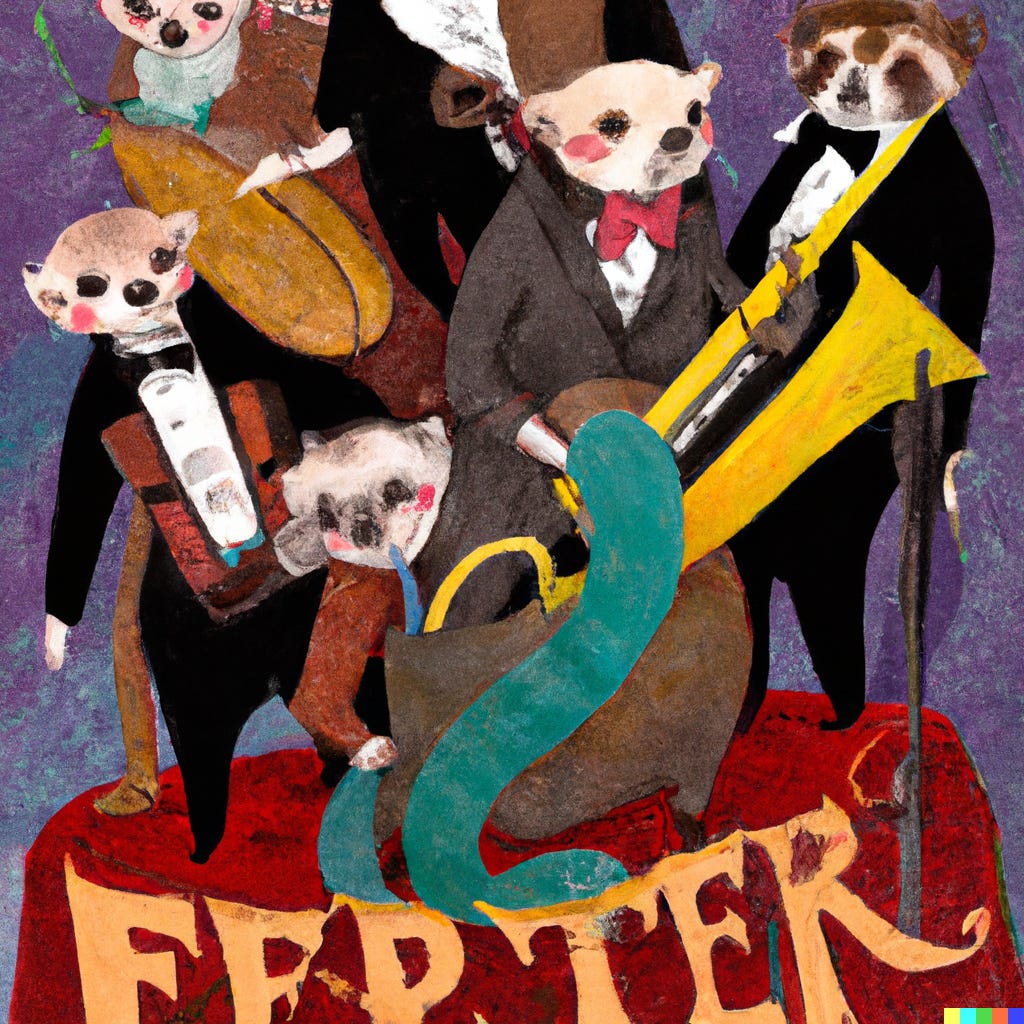
The ferret’s visual systems began to invade the space left over by the now defunct auditory cortex, and regions of the auditory cortex began responding to visual stimuli, like the orientation of lines.
What the hell, man? You told me that we weren’t blank slates!
Dehaene doesn’t believe this is evidence that we are blank slates. Neither does Sur, who argues that this transmutation of auditory cortical material into visual cortex is, basically, bad. The visual maps in the auditory cortex are thought to be much less accurate than the equivalent circuitry would be in the visual cortex. How were they less accurate? Responses in the MGN were slower, and responsive to much larger patches of light than their specialised companions in the lateral geniculate nucleus, which is part of the ‘traditional’ circuitry for processing visual information.
We should note that the reason that ferrets were selected was not so I could make excellent musical ferret puns, but because ferrets have immature projections away from the retina at birth. If they had chosen cats, this wouldn’t have worked, because their retinal projections are more developed when they’re born. Therefore, the idea that humans are blank slates at birth also depends on how developed human cortical circuitry is at birth.
So, how mature are human retinal projections at birth? Well, they do develop in utero, but they remain somewhat inchoate at birth. The primary auditory cortex is structurally mature after 28 weeks of gestation. This doesn’t mean that we don’t learn from our environments, simply that our learning is heavily shaped by structures that we already have when we’re born. We’ll discuss this idea more in Part 2 of this post (but feel free to get excited now!).
All of your neural circuitry is thought to be something like this. It can adapt, and there is evidence of neural plasticity all over the brain, but it’s much happier doing what it was designed to do, genetically speaking. But your synapses are very plastic where it matters: in those final millimetres of synapse and neuron that are malleable in response to the environment.
Dehaene:
The brain can use synaptic plasticity to self-organise: it first generates activity patterns purely from within, in the absence of any input from the environment, and uses those activity patterns, in combination with synaptic plasticity, to wire its circuits. In utero, even before they receive any sensory input, the brain, the muscles, and even the retina already exhibit spontaneous activity (this is why fetuses move in the womb). Neurons are excitable cells: they can fire off spontaneously, and their action potentials self-organise into massive waves that travel through brain tissue. Even in the womb, random waves of neuronal spikes flow through the fetus’s retinas, and upon reaching the cortex, although they do not carry any visual information in the strict sense of the term, these waves help organise the cortical visual maps. Even after birth, random neuronal firing unrelated to sensory inputs continues to flow through the cortex.
This idea of spontaneous neural activity is interesting, because it provides an initial impetus for Bayesian theories of the brain. As I discussed in my post on Bayesian ideas of neural processing, mental models are thought to be a combination of top-down predictions (priors) and bottom-up prediction errors, which are combined into new (posterior) predictions. One of the initial quibbles that people had with this theory was what I’ll call the problem of the First Prior.
The First Prior problem just poses the problem of the origin story; Spiderman came from a mutant spider; Batman came from watching his parents get shot; where do priors come from?
Spontaneous activity offers something of a solution. While the fetus is chilling out in the womb, waves of activity flow through the fetus’ retinas, and despite the fact they carry no visual data, the brain begins to create cortical visual maps. As the retina matures, these visual maps finally have some material to test their ideas on.
Similarly, in Anton Chekhov’s story The Bet, a man is locked away, reading books for twenty years, until he is finally loosed onto the world, and can finally test the models of the world that he learned in his books:
For fifteen years I have been intently studying earthly life. It is true I have not seen the earth nor men, but in your books I have drunk fragrant wine, I have sung songs, I have hunted stags and wild boars in the forests, have loved women ... Beauties as ethereal as clouds, created by the magic of your poets and geniuses, have visited me at night, and have whispered in my ears wonderful tales that have set my brain in a whirl.
He runs off into the world, to live and to experience all the things he has only read of.
DEHAENE TRAVELS TO THE LAND OF THE FOUR PILLARS
Dehaene chooses four pillars that should explain the four key elements of learning. These are attention, active engagement, error feedback, and consolidation. We’ll look at them in turn, and how they explain integral parts of the learning process.
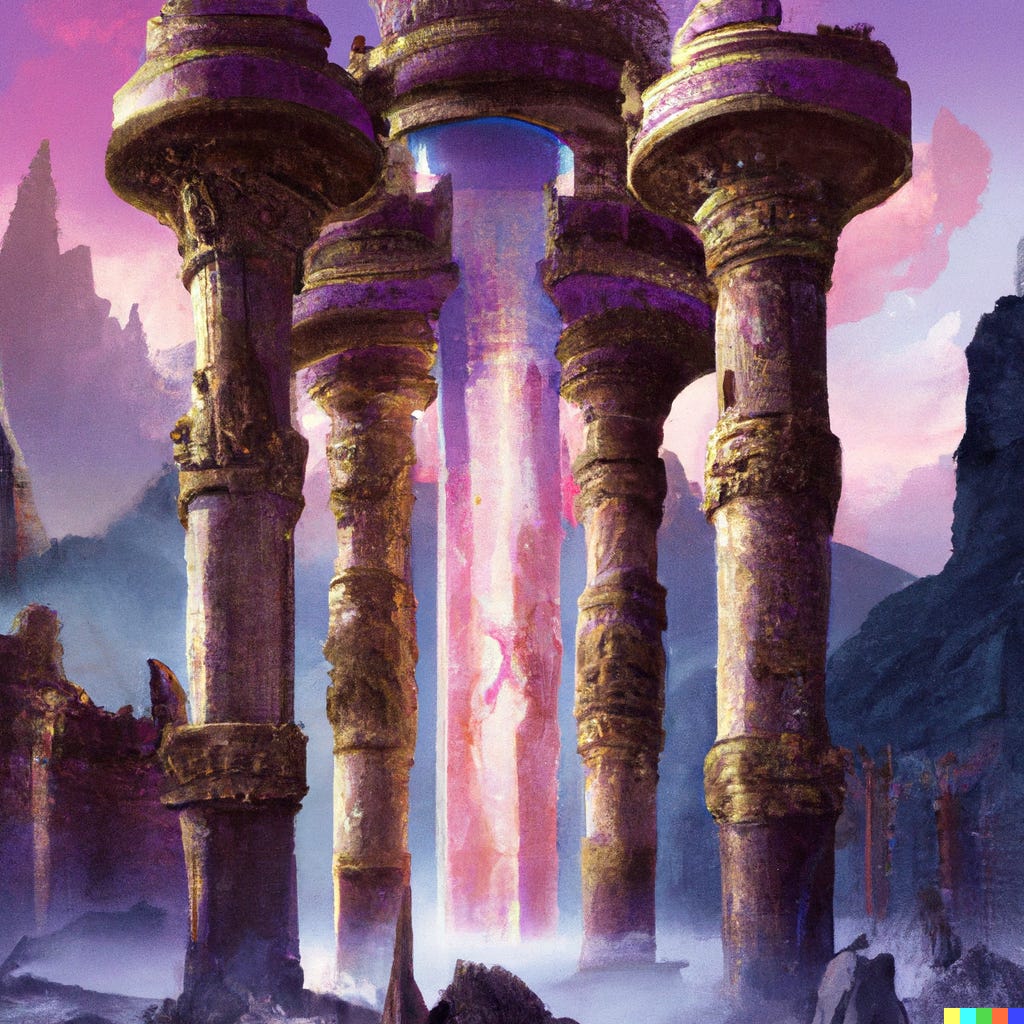
These are Dehaene’s four pillars of learning. Let’s dig in, one at a time.
ATTENTION
Dehaene asks a question I hadn’t really considered before: “why evolve attention?” I suppose in biological sciences you can almost always ask the question “why evolve this thing?” and it’ll be somewhat interesting. Perhaps I’m very naive and real biologists are very bored of these questions, but given I came across a paper this week on why giraffes evolved such weird recurrent laryngeal nerves, I doubt it.
Anyway, without attention we would totally overloaded by information. Attention picks salient parts of a scene, or a problem, or a book review, and flags them for learning to take place.
It’s-a-gooda-mechanism. Throughout the book, Dehaene compares and contrasts what we know of the brain with what we known of neural networks in machine learning. The two fields have heavy overlap, and the metaphors and ideas of both fields bleed into each other. I won’t discuss the machine learning side at great length in this post, but it’s useful to note that ideas about learning are frequently testing using neural networks and their ilk. Accordingly, if you add attention into artificial neural networks (ANNs), it aligns processing onto the most relevant parts of a problem, and this makes ANNs run much faster.
Have you ever noticed that you don’t learn much when you’re trying to read a book review and watch Severance and listen to the new Django Django album and argue with your mate about which restaurant you’re going to order from? This is because learning relies directly on attention.
Michael Posner describes three major attention systems:
Alerting, which indicates when to attend, and adapts our level of vigilance.
Orienting, which signals what to attend to, and amplifies any object of interest.
Executive attention, which decides how to process the attended information, selects the processes that are relevant to a given task, and controls their execution.
System Number 1, alerting, is a direct response to threat. This is a ‘I’m walking down a street and someone pulled a knife on me’ type deal. Or ‘I just bought me and my boyfriend two tickets to Barcelona and now he’s breaking up with me’. The whole cortex lights up, trigged by subcortical nuclei. There are massive and diffuse releases of key neurotransmitters; serotonin, acetylcholine and dopamine.
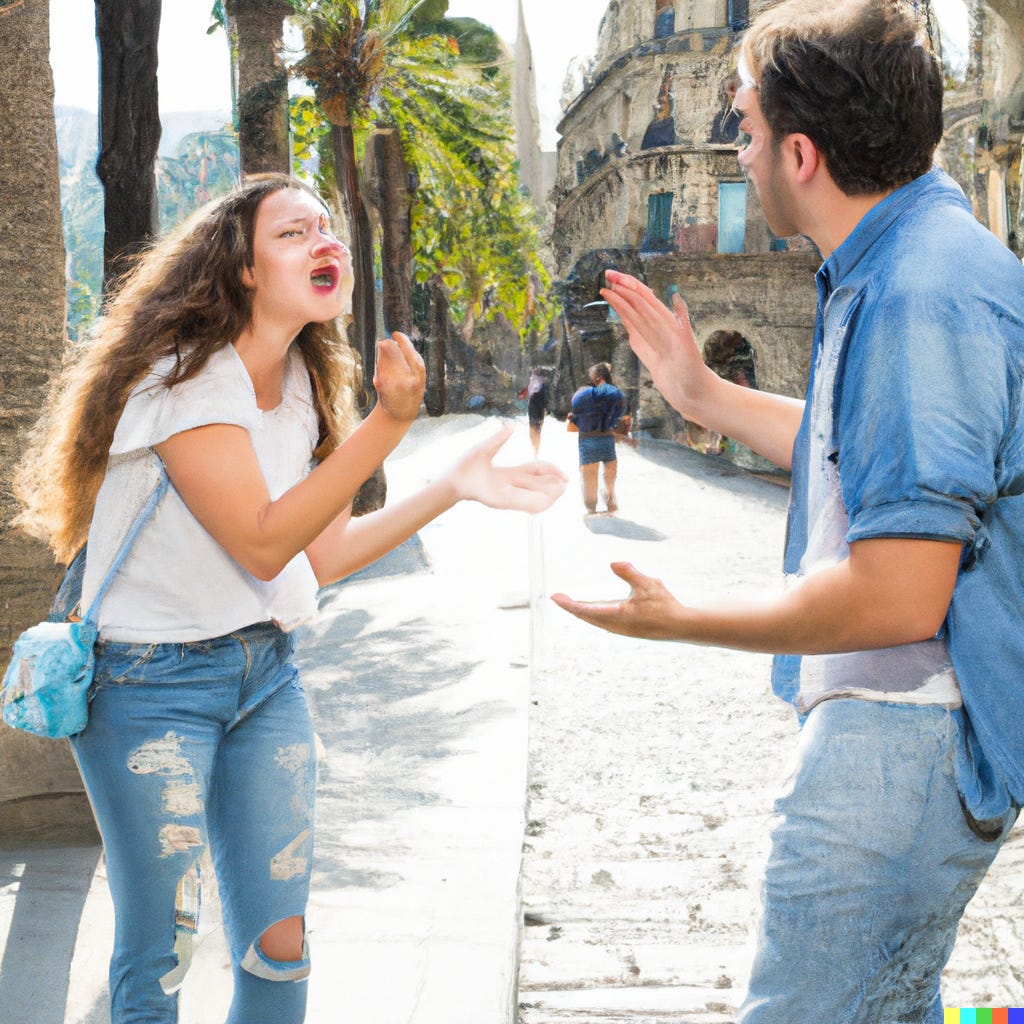
Researchers refer to a “now print” signal; this is the cortex saying, remember everything NOW because I’m MAD as hell and I ain’t going to TAKE it no more. This is a ‘I don’t want to be in this place, get me out, get me out’ type reaction.
You can electrically stimulate those same subcortical nuclei that fuck-you-up in mice, and any neurons currently active get heavily amplified. Anything else gets de-emphasised, and the mice get much worse at processing the de-emphasised information, in favour of the informations which seems to be currently causing their stress. Active mice neurons scream ‘I’m active and this scenario is bad so I must be a bad neuron’, and the mice also start listening to the Chemical Brothers’ Mad As Hell way more.
Attention seems to work by a form of bias. At any given moment, hundreds of sensory inputs compete for priority in the brain, and the attention mechanism selects a specific set of these inputs. To suppress unwanted activity, the brain seems to swamp areas that are not necessary right now with slow waves in the alpha frequency band which prevent those areas from developing coherent neural activity.
The classic example of this is the gorilla experiment, where you’re so busy counting the passes between basketball players that you don’t notice the gorilla walking through. Obviously, if you go away and watch that video, you will notice the gorilla, because a) you’re not invested in the task, and b) you know a gorilla is about to walk through.
This experiment does replicate however. Researchers did something similar with a driving simulator, where you had to brake for certain colours and not others, and if motorcyclists not wearing the correct colours came past, people crashed into them. This all suggests that our attentional set can tune into colour, and count or discount that effectively. Is it just colour? It’s unlikely, because the structure of our attentional sets seems fairly complex.
Lower levels of the visual cortex process simpler shapes and forms. Higher levels combine those lower perceptions with ideas of how things work, and create the complex multisensory experience that you have everyday.
A possibility is that colour can be discounted at early levels of the visual hierarchy. Complex regions issue a command saying that we’ll discount some stimuli in favour of upweighting other stimuli, because we’re doing this random counting task in order to get a marshmellow, and by gum we want that marshmellow. Does that make sense?
In the gorilla video, an important element of ignoring the gorilla is the fact that the gorilla is dressed in black, as one of the irrelevant stimuli (the team whose passes you are not counting), and is human shaped.
If a real gorilla entered, people would probably notice the gorilla, because even if gorillas are black, they are usually not human-shaped, except of course in the below drawing of French explorer Paul Du Chaillu with a human-shaped gorilla.
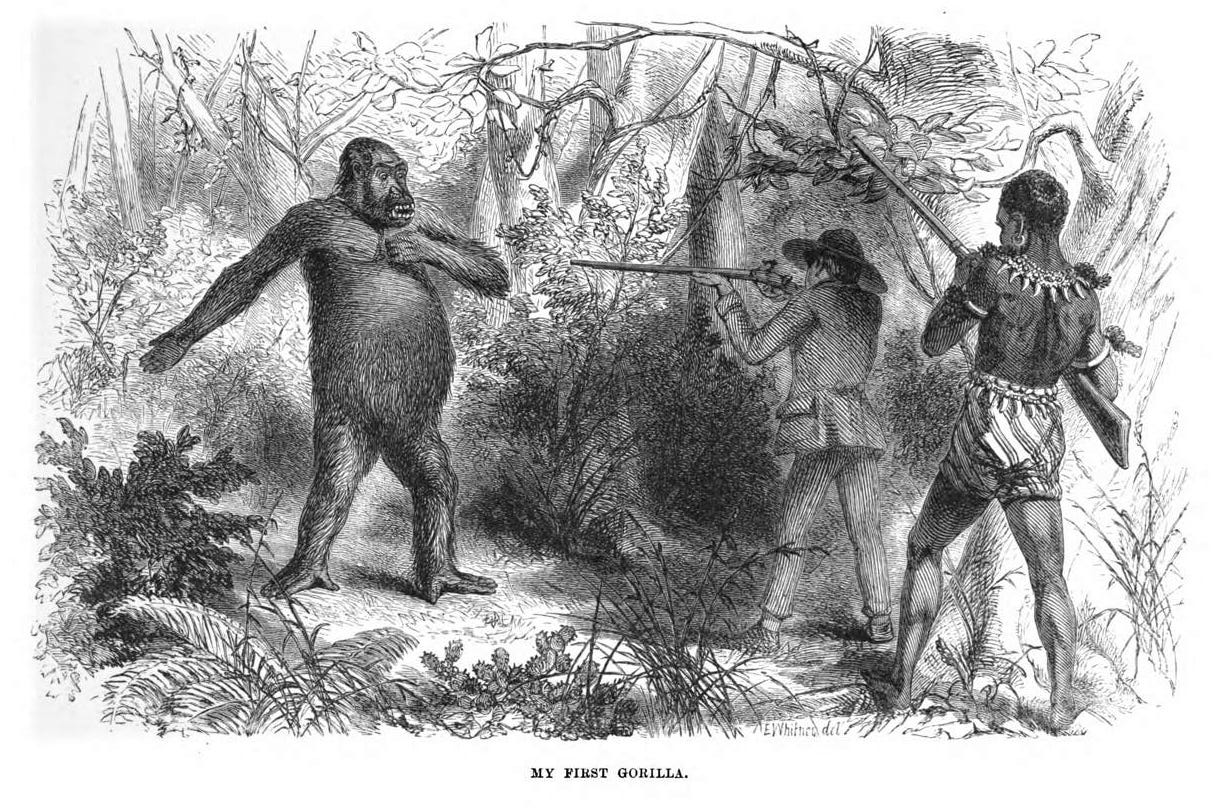
Attention to different things utilises different circuits, with different advantages and drawbacks. In one study, paying attention to letters allowed participants to learn new words and shapes in a made up language, while paying attention to the whole words did not. Incidentally, action video games seem to offer an effective form of attention training (emphasis mine):
A review of the aspects of attention enhanced in action game players suggests there are changes in the mechanisms that control attention allocation and its efficiency (Hubert-Wallander et al., 2010)... …Moving distractors were found to elicit lesser activation of the visual motion-sensitive area (MT/MST) in gamers as compared to non-gamers, suggestive of a better early filtering of irrelevant information in gamers. As expected, a fronto-parietal network of areas showed greater recruitment as attentional demands increased in non-gamers. In contrast, gamers barely engaged this network as attentional demands increased. This reduced activity in the fronto-parietal network that is hypothesized to control the flexible allocation of top-down attention is compatible with the proposal that action game players may allocate attentional resources more automatically.
I’m so glad I spent all those years no-scoping people with a Barrett 50. cal in Call of Duty, they’ve really paid off for me because I have the best attention span ever and it really lets me focus on things I’m writ
There is also a “central bottleneck” of attention. This means you can only process one stream of information at any one time, and accordingly, you are unaware of the other things that you are not currently paying attention to, like gorillas or motorbikes. You should also be unaware of this limitation.
And why would you be aware of that limitation? It would be weird to have awareness of your unawareness.
Dehaene:
A basic experiment illustrates this point: Give someone two very simple tasks - for example, pressing a key with the left hand whenever they hear a high-pitched sound, and pressing another key with the right hand if they see the letter Y. When both targets occur simultaneously or in close succession, the person performs the first task at normal speed, but the execution of the second task is considerably slowed down, in direct proportion to the time spent making the first decision. In other words, the first task delays the second: while our global workspace is busy with the first decision, the second one has to wait. And the lag is huge: it easily reaches a few hundred milliseconds. If you are too concentrated on the first task, you may even miss the second task entirely.
Remarkably, however, none of us is aware of this large dual-task delay - because, by definition, we cannot be aware of information before it enters our conscious workspace. While the first stimulus gets consciously processed, the second one has to wait outside the door, until the global workspace is free - but we have no introspection of that waiting time, and if asked about it, we think that the second stimulus appeared exactly when we were finished with the first, and that we processed it at a normal speed.1
The only exceptions are tasks where people have learned very high levels of automaticity. For instance, very good pianists can have a conversation while they play a piece they know well, but these are exceptions, and still debated. Regardless, I doubt very good pianists can improvise while they talk, or have a complex conversation.
Dehaene notes that this central bottleneck is more critical for children, whose attentional resources are undeveloped - they haven’t yet wasted their youth shooting virtual zombies to train them. So, doing one thing at a time is imperative for learning. Even an overly decorated classroom can distract children and prevent them from concentrating. Children allowed to use their phones in class perform worse when tested on any material covered in that class, even months later.
ACTIVE ENGAGEMENT
Any learning has to be active. What does Dehaene mean by this? You need to be generating mental models of the world, and then actively testing them. Remember above, where I talked about babies chucking their lunch around? That involves generating a mental model - lunch will take this path if I throw it here, and testing it - I threw lunch in that spot, and it scattered in a way I didn’t expect.
Dehaene did an experiment where he presented 60 words to three groups of children. He then asks the first group whether the words’ letters are uppercase or lowercase, the second group whether they rhyme with “chair”, and the third group whether they are animals.
Which group remembers the words best?
The first group remembered 33% of the words, the second remembered 52% and the third group remembered 75%. Why? Dehaene suggests that the third group were processing words at the level of meaning, rather than superficial details about the word.
The American psychologist Henry Roediger, who wrote Make It Stick (with co-authors Peter Brown and Mark McDaniel) suggests that:
“Making learning conditions more difficult, thus requiring students to engage more cognitive effort, often leads to enhanced retention.”
Deeper processing like this is thought to be embedded into memory better because it activates areas of the prefrontal cortex associated with conscious word processing, and these areas then form loops with the hippocampus, which stores information in the form of explicit episodic memories.
Structured, active environments engender learning.
Two sets of students are learning about angular momentum and angular torque. One group gets given a bicycle wheel and 10 minutes to experiment, one group gets 10 minutes of verbal explanations. The experimenting group learns much more effectively.
I remember once during my undergraduate degree, I went to a seminar with prominent members of the faculty, who discussed the effectiveness of their teaching strategies. At one point a student tried to critique the main form of teaching on the course, which was around eight hours of lectures a week, often presented together in large blocks early in the morning. The professorial staff were not happy with this critique, and all laid into this poor student (eight or so distinguished lecturers versus a twenty-year old undergrad is not a fair fight), and essentially argued that this poor student was lazy.
I didn’t know the guy, so he may well have been lazy, but he was also right. For teaching, long lectures are pretty bad. Being talked to for an hour doesn’t put knowledge into the brain. The problem may come from an asymmetry of effectiveness. Lectures are great strategies for cementing your understanding of a topic - if you are giving the lecture. Teaching is a really effective way of learning, as Richard Feynman was fond of pointing out. But for those on the receiving end of the lecture, especially if they’re in a big group as opposed to being taught 1-to-1, this was a wildly inefficient use of their time:
The studies analyzed here document that active learning leads to increases in examination performance that would raise average grades by a half a letter, and that failure rates under traditional lecturing increase by 55% over the rates observed under active learning. The analysis supports theory claiming that calls to increase the number of students receiving STEM degrees could be answered, at least in part, by abandoning traditional lecturing in favor of active learning.
What are good active strategies? What do we instead? Yeah. The reason that lectures haven’t been shuffled out is probably because there isn’t an obvious replacement that can be systematically implemented.
There are ideas that float around the internet: Kai Chang writes of one lecturer who would insert a lie into every lecture in order to keep his students guessing, which is an ingenious yet subtle strategy. It also requires the lecturer to be something of a genius themselves, because coming up with effective lies, especially in advanced learning environments, is very difficult. One proposal, put forward by Gwern, involves using the burgeoning capabilities of natural language processing to simulate journal articles from a certain point, and have students try to determine when this point began.
These ideas, while interesting, are all aimed at higher levels of education. Activity at lower levels can simply involve allowing students to experiment whilst being given clear rigorous instructions. That ‘whilst’ is critical. Just allowing students to experiment is a bad idea, and Dehaene is keen to work against ‘discovery learning’ ideas. Briefly, these are descended from Rousseau, who wrote:
Teach your student to observe the phenomena of nature and you will soon rouse his curiosity; but if you want his curiosity to grow, do not be in too great a hurry to satisfy it. Lay the problems before him and let him solve them himself.
This piece by Simon Sarris makes a similar argument in the modern day - that children need to be given room to do things and experiment with ideas in open environments that school does not provide.
Dehaene says that this doesn’t work. It’s too hard for students to derive complicated theories about how the world works for themselves. There’s a reason humans suffered for thousands of years trying to create them. Unless your child is literally Gauss, asking them to derive mathematical solutions from scratch isn’t going to work. Even bright children who can derive such solutions often end up performing worse later on problems than those who were taught how to do it and then went away and did it.
Don’t get this wrong - the active exploration stuff is good, but it should be combined with information about the structure of the environment that you’re learning in.
Hang on, what about all those self-taught programmers?
Education is almost certainly a high variability environment, so there are probably some people who can manage it. It’s also likely possible with enough brute force and motivation to do most educational strategies and they’ll work. In this talk, John Hattie makes the point that there are almost no educational interventions that do nothing. Any teaching at all will work, as long as they don’t involve eight hours of throwing darts at children - and even then, the kids will probably get good at dodging darts. But different techniques will vary massively in effectiveness.
Dehaene gives a personal account of his experience with discovery learning:
I directly experienced the birth of the personal home computer - I was fifteen years old when my father bought us a Tandy TRS-80 with sixteen kilobytes of memory and 48-by-128-pixel graphics. Like others of my generation, I learned to code in the programming language BASIC without a teacher or a class - although I was not alone: my brother and I devoured all the magazines, books, and examples we could get our hands on. I eventually became a reasonably effective programmer… but when I entered a master’s program in computer science, I became aware of the enormity of my shortcomings: I had been tinkering all this time without understanding the deep, logical structure of the programs, nor the proper practices that made them clear and legible. And this is perhaps the worst effect of discovery learning: it leaves students under the illusion that they have mastered a certain topic, without ever giving them the means to access the deeper concepts of a discipline.
One question that arises from this argument is whether such teaching undermines students way down the line, when they need to generate their own original ideas. This feels hard to design studies for, and the schools and creativity debate is cluttered and confusing. Nonetheless, the bulk of work usually requires concrete, known, skillsets and the ability to develop those skillsets.
So, combining clear, structured advice along with experimentation in confined scenarios should be better for the vast majority of cases. I’m sure plenty of teachers already do this, but some obviously don’t. Towards the end of the book, Dehaene bemoans the lack of education for teachers about the effectiveness of different types of teaching, and cites this Richard Mayer quote:
[The best success is achieved by] methods of instruction that involve cognitive activity rather than behavioural activity, instructional guidance rather than pure discovery, and curricular focus rather than unstructured exploration.
Dehaene cites the example of current Montessori teaching methods, which apparently have three parts: (1) outline a series of activities, (2) have the teachers clearly explain their purpose, (3) allow the children room to experiment.
There is a long section on the importance of stimulating curiosity. Curious children outperform children who don’t give a fuck in tests, even if not giving a fuck is way cooler and gets you all the chicks. In kindergarten, the most curious students are also those who do better in reading and math. The degree of curiosity you feel correlates tightly with the activity of the nucleus accumbens and ventral tegmental area, two essential regions of the dopamine brain circuit. When curiosity is higher, your memory is stronger, and memories get better the more curious you are. Learning gets so strong that you start remembering incidental details better - the face of the person who taught you a fact, say.
And children are naturally curious. Once they’ve learned to talk, they often brim with questions, including those fascinating “Why? Why? Why?” strings which infinitely recurse into your frustration and anger as you realise you don’t and have never know the true reason why bees buzz or the solar system formed. Then at some point, children stop being curious. Dehaene suggests three possibilities, which I’ve summarised:
Curiosity dwindles naturally. As learning progresses, expected gains fall. The better we master a field, the less it can offer us. To maintain curiosity, schools must therefore provide children’s brains with stimulants that match their intelligence, which is not always the case. Advanced students are not provided with advanced enough materials. Struggling students may learn that they are not good at learning, and that they are incapable of learning maths and history etc. When children are discouraged, the key is to offer them problems that are tailored to their level.
Punishment. Saying for instance a question is stupid deactivates the child’s curiosity circuit. Repeated punishment leads to learned helplessness, a paralysis which inhibits learning in animals.
Teaching. Teaching can kill curiosity. Show a group of kids a wacky device with loads of functions, and let them explore it, and they go nuts. Do the same but have a teacher explain it, and the children assume that the teacher has introduced most of the cool functions, and so there is no need to explore the device. Students also factor in the teacher’s style of instruction - if teachers always make lengthy demonstrations, the students lose curiosity, because they know that that teacher has likely exhausted all the possibilities.
How do you sustain a child’s curiosity? There is a bell curve of “interestingness”, a Goldilocks zone of complexity, a twilight zone of fascination. Stimuli need to be just interesting enough. The key is what psychologists call ‘desirable difficulties’. Anything hard enough to be challenging, but not so difficult as to prevent progress.
ERROR FEEDBACK
The first two pillars are more difficult to measure. That hasn’t stopped people measuring them, but error feedback is a simpler metric to assess. We give some people feedback, and we don’t give some other people feedback. Who does better? The people with feedback.
John Hattie ran multiple meta-analyses on the effect sizes of various educational interventions. They’re broken down quite substantially, but here’s the fun barometer version:
Feedback gets an effect size of about 0.7 standard deviations, i.e., which is usually classified as a ‘medium-large’ effect. If you’d like to see a complete list with numbers instead of the colourful barometer, there’s an image which compiles all the numbers.
There are important caveats in the way feedback has to be structured:
Feedback at the process level is most beneficial when it helps students reject erroneous hypotheses and provides cues to directions for searching and strategizing. Such cues sensitize students to the competence or strategy information in a task or situation. Feedback at the self or personal level (usually praise), on the other hand, is rarely effective. Praise is rarely directed at addressing the three feedback questions and so is ineffective in enhancing learning. When feedback draws attention to the self, students try to avoid the risks involved in tackling challenging assignments, to minimize effort, and have a high fear of failure (Black & Wiliam, 1998) to minimize the risk to the self.
There’s a lot to say about feedback, but in this section I’d rather focus on the idea of feedback as a form of surprise. Dehaene discusses a model put forward by Robert Rescorla and Allan Wagner, whose guiding principle is that “Organisms only learn when events violate their expectations”.
How does that work? It’s an way of framing the Bayesian models of mind - everything is, in this world:
The brain generates a prediction by computing a weighted sum of its sensory inputs.
It then calculates the difference between this prediction and the actual stimulus it receives: this is the prediction error, a fundamental concept of the theory, which measures the degree of surprise associated with each stimulus.
The brain then uses this surprise signal to correct its internal representation: the internal model changes in direct proportion to both the strength of the stimulus and the value of the prediction error. The rule is such that it guarantees that the next prediction will be closer to reality.
What were the alternative models of learning?
Behaviourists used to fill up their tank with associationist views, where they assumed organisms just recorded stimuli and developed responses to them in a passive way. For example, if you just saw two things together, you might learn that they are connected. This isn’t how the brain works, and we can prove it.
Dehaene:
Forward blocking provides one of the most spectacular refutations of the associationist view. In blocking experiments, an animal is given two sensory clues, say a bell and a light, both of which predict the imminent arrival of food. The trick is to present them sequentially. We start with the light: the animal learns that whenever the light is on, it predicts the arrival of food. Only then do we introduce dual trials where both light and bell predict food. Finally, we test the effect of the bell alone. Surprise: it has no effect whatsoever! Upon hearing the bell, the animal does not salivate; it seems utterly oblivious to the repeated association between the bell and the food reward. What happened?
The finding is incompatible with associationism, but it fits perfectly with the Rescorla-Wagner theory. The key idea is that the acquisition of the first association (light and food) blocked the second one (bell and food). Why? Because the prediction based on light alone suffices to explain everything. The animal already knows that the light predicts the food, so its brain does not generate any prediction error during the second part of the test, where the light and the bell together predict the food. Zero error, zero learning - and thus the dog does not acquire any knowledge of the association between the sound and the food. Whichever rule is learned first blocks the learning of the second.
Similarly, whenever babies perceive an event as impossible or improbable, learning mechanisms are enhanced. If babies see an object mysteriously pass through a table - yeah, I do multiple callbacks - they stare at this impossible scene, and subsequently better remember the sound that the object made, or even the verb that the adult used to describe the action.
But how do you know whether 11 month olds have learned anything?
Wow, I literally had the exact same question. Stahl and Ferguson explain:
For each infant we calculated a learning score by determining the proportion of time that infants looked at the target object (relative to the new distractor object) during the baseline, then subtracting this value from the proportion of time they looked at the target object during the mapping test, when the taught sound played. If infants had successfully learned the object-sound mapping, they should increase the proportion of time they looked at the target object when the sound played; such auditory-visual “matching” is the pattern typically observed in studies of infants’ mapping abilities (21).
Or if you give babies the table-busting toy, they play with it for longer.
Everything in the cortex is thought to be running error detection software. It’s easiest to show this in the auditory cortex, so Dehaene chooses that cortex. Great choice, Stanislas. There’s a special type of signal that gets fired out in response to an “error”, known as a Mismatch negativity response.
Dehaene writes out notes, and then shows you how errors are associated with those notes. But we’re online! I can play you music! Terrible, terrible music!
Let’s say you just hear the note C a load of times, rendered here by one of my favourite virtual instruments, Red Delicious:
The pattern is so predictable that it becomes boring. The auditory cortex rapidly diminishes the response, a process known as ‘adaptation’. If I played this to you for three minutes, you’d probably a) lose your mind, or the more likely b) tune it into the background and think about what you want to think about, probably pie because you like pie.
What if I vary the notes?
Now we get to the good part. I don’t mean the above ‘music’, that’s still pretty bad. But it should have also been firing error messages, and not for the reason you might assume that it is.
The notes I was playing for you there go C C G G (x4). When you hear the G, lower levels of the auditory system are mildly surprised - G is a different note from C. But a higher level circuit is surprised for a different reason, and these systems are hierarchical, so the higher level surprise matters more.
Can you figure out why?
This more natural continuation of those notes might help:
Higher level predictive circuits were expecting to hear that slamming track, and they didn’t. They heard C C G G C C G G, and they were confused. Then they probably realised I was doing something different.
Real musicians learn this, whether explicitly or intuitively. Then they play things that hint at patterns you know:
Yeah, real musicians definitely do stuff like that. That’s why I’m totally a real musician. They might resolve into patterns you know, or deliberately tantalise you by leaving them just out of reach. Or they might do whatever jazz is. Obviously, you can establish these patterns within the confine of any song, everyone isn’t just riffing on Twinkle Twinkle Little Star.
This happens a lot in musical theatre where people pen verses which go like:
I am so done with that mad girl,
I wanna throw her in a deep ditch,
But she still sets my heart awhirl,
If only she wasn’t such a bad person
What? What were you expecting the last word to be?
Dehaene:
The auditory cortex seems to perform a simple calculation: it uses the recent past to predict the future. As soon as a note or a group of notes repeats, this region concludes that it will continue to do so in the future. This is useful because it keeps us from paying attention to boring, predictable signals. Any sound that repeats is squashed at the input side, because its incoming activity is canceled by an accurate prediction. As long as the input sensory signal matches the prediction that the brain generates, the difference is zero, and no error signal gets propagated to higher-level brain regions.
Subtracting the prediction shuts down the incoming inputs - but only as long as they are predictable. Any sound that violates our brain’s expectations, on the contrary, is amplified. Thus, the simple circuit of the auditory cortex acts as a filter: it transmits to the higher levels of the cortex only by the surprising and unpredictable information which it cannot explain by itself.
All aspects of the cortex are doing this, according to current theories. Visual systems, tactile systems, olfactory systems, and they’re likely combining information at high levels of the hierarchy if perception in any given system isn’t enough.
Enough about surprise. I want to hear about how good current feedback is. What about existing forms of feedback, like grades? Are they useful?
Not that useful, apparently. It’s important for children to be able to correlate their grades with the mistakes they make, otherwise the feedback loop of the grade offers little. Usually, there’s a significant time delay between a mistake and a grade, which means that learning doesn’t really occur. Grades often get used as punishments - and adolescents usually respond better to rewards than to punishments - and can engender things like mathematics anxiety.
Maths anxiety, by the way, is a quantifiable syndrome, and children who suffer from it show activation in the pain and fear circuits, including the amygdala. Stress and anxiety generally destroy the ability to learn. In mice, fear conditioning ossifies neuronal plasticity - if the animal is traumatised by random, unpredictable shocks, synapses become immobile. Returning to a fear-free environment allows synapses to become plastic again.
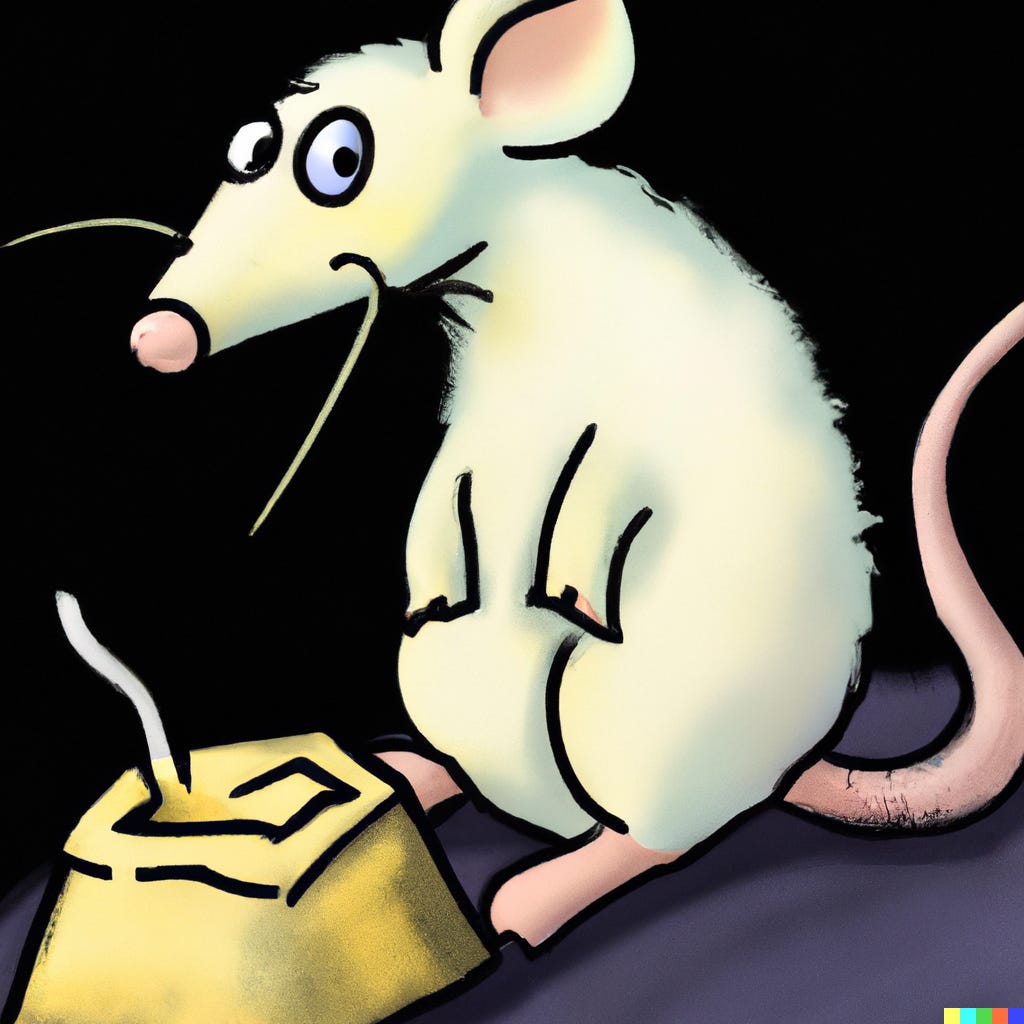
I’ve covered this before, but the best way to learn things is testing, and particularly regular testing. This is known as “retrieval practice”. It maximises long-term learning, because it makes it very clear what you know and what you don’t know. Often we know things in a somewhat blurry way, usually in areas where we have learned a topic and not reviewed it properly, but because we did learn the topic area, we think we know it well.
The human brain is good at deceiving itself as to how much it actually knows, especially when it used to have a certain set of knowledge. Things like cramming work for a short time, but exchange knowledge now for knowledge later. This then provides the brain with the deceptive idea that it retains that knowledge. It doesn’t. The key part of any test is the effort made to retrieve information, and the immediate, quick feedback you receive. The relationship between the test and the grade is superfluous.
Spacing is critical. Learning increases by a factor of three when you review at regular intervals as opposed to learning everything at once. Why does spacing work? It may be that problems, when mashed into a tight time window, reduce brain activity due to adaptation or habituation.
According to Hal Pashler, the optimal spacing intervals are about 20% of the time period you need to recall things over. If you want to remember something after about 10 months, you should learn it, then review it after about 2 months. This is a simple form of a learning algorithm, and I doubt it’s particularly effective. Most spaced repetition programs will show you information much more than this. But regardless of the details of any optimal learning algorithm, textbooks, which show you one block of information then another block, are often poorly designed. Problems should be continually mashed together, as this also improves retention. (1, 2)
Feedback improves memory even on correct trials, so testing yourself isn’t purely about improving the memory of stuff you don’t know, it also helps with the things that you do know.
Are there limits to memory storage? The constraint is in the access to memories, rather than in the amount that can be stored. You can store an almost unlimited amount of things, but as we get older, our access mechanisms seem to have problems. This is also why its usually better to learn things at a greater depth, as opposed to knowing a lot of things at a shallow level, because retrieval usually triggers representations of other related objects.
CONSOLIDATION
Even when a skill is mastered, we continue to overlearn it. As we learn things, they move from intensive parietal and frontal-lobe regions to more automatic areas. Automatisation allows the brain to do more, because it frees the central bottleneck.
Sleep critically causes extra learning - without any extra training, cognitive and motor performance improved after a period of sleep. The amount of learning gain corresponds to the quality of sleep, and fascinatingly, the need for sleep also depends on the amount of stimulation and learning that occurred during the previous day. Duration and depth of sleep predict a person’s performance when waking.
In animals, a gene involved in cerebral plasticity, zif-268, increases its expression in the hippocampus and cortex during REM sleep, and specifically when the animals were exposed to an enriched environment - the increased stimulation led to a surge in brain activity.
Hippocampal reactivation during sleep seems to allow automatisation. The more a neuron reactivates during the night, the more learning that occurs. Similar processes occur for humans:
During sleep, brain activity oscillates spontaneously at a slow frequency on the order of forty to fifty cycles per minute. By giving the brain a small additional kick at just the right frequency, we can make these rhythms resonate and increase their intensity - a bit like when we push a swing at just the right moments, until it oscillates with a huge amplitude.
German sleep scientist Jan Born did precisely this in two different ways: by passing tiny currents through the skull, and by simply playing a sound synchronised with the brain waves of the sleeper. Whether electrified or soothed by the sound of the waves, the sleeping person’s brain was carried away by this irresistible rhythm and produced significantly more slow waves characteristic of deep sleep. In both cases, on the following day, this resonance led to a stronger consolidation of learning.
If you read the above quote and thought, wow, that could be a business, some people have already made some of these headsets, but I don’t see why this couldn’t be a product for mass-market as opposed to just clinical trials.
If you learn something during the day while smelling roses strongly, and you then fall asleep and then we fill the room you’re sleeping in with the scent of roses, there’s some evidence that it strengthens the memories of the learning (as opposed to being exposed to a different smell). A similar tactic works for sound. None of this means you can learn fresh information in your sleep. You can’t. Throw anyway the magic $50 VHS tape you bought from that shady man in the market who claimed it would teach you Spanish. Sleep strengthens recall, not learning.
But it may also help with breakthroughs. The most famous example of this is August Kekule von Stradonitz who dreamed up the structure of benzene in his sleep. Benzene is unusually structured - its six carbon atoms form a closed loop, like a snake biting its tail:
![Kekulé's 1872 modification of his 1865 theory, illustrating rapid alternation of double bonds[note 1] Kekulé's 1872 modification of his 1865 theory, illustrating rapid alternation of double bonds[note 1]](https://substackcdn.com/image/fetch/w_1456,c_limit,f_auto,q_auto:good,fl_progressive:steep/https%3A%2F%2Fsubstack-post-media.s3.amazonaws.com%2Fpublic%2Fimages%2F48e9b9b1-41ac-4348-a9c9-60ed1428c68c_1081x375.png)
Kekule:
Again the atoms were gamboling before my eyes … My mental eye, rendered more acute by repeated visions of this kind, could now distinguish larger structures of manifold conformation; long rows sometimes more closely fitted together, all twining and twisting in snake-like motion. But look! What was that? One of the snakes had seized hold of its own tail, and the form whirled mockingly before my eyes.
Let us learn to dream, gentlemen, and then perhaps we shall learn the truth.
This may go a little too far. But a study from Wagner et al. suggests that sleep doesn’t simply encode data, it encodes it in a more abstract and generalised way, which helps to find solutions.
Dehaene:
During the day, these researchers taught volunteers a complex algorithm, which required applying a series of calculations to a given number. However, unbeknownst to the participants, the problem contained a hidden shortcut, a trick that cut the calculation time by a large amount. Before going to sleep, very few subjects had figured it out.
However, a good night’s sleep doubled the number of participants who discovered the shortcut, while those who were prevented from sleeping never experienced such a eureka moment. Moreover, the results were the same regardless of the time of day at which participants were tested. Thus, elapsed time was not the determining factor: only sleep led to genuine insight.
Why does this happen? Dehaene suggests that the combination of bottom-up and top-down models is tilted towards corrections during the day, the bottom-up data being checked frequently. This allows us to check and refine parameters of the model. At night, the balance flips the other way.
Dreams can be thought of an expression of this process. If you have no bottom-up sensory data to test theories against, then the top-down data is free, essentially, to hallucinate phenomena. This shouldn’t in theory, be random, but instead a set of targeted experiments on data.
Many learning algorithms lack data. Things like ChatGPT, everyone’s favourite algorithm, require vast amounts of input before they generate useful output. Our brains obviously can’t access training data in this same way, so they use the generative elements of the model to create a new set of images, and train themselves on that. Whether this is an accurate explainer of dreams remains uncertain, but its an interesting theory.
We do know that sleep functions are probably more effective in humans than in other primates, and in turn that children sleep more efficiently than adults.
Adolescents struggle to get up, likely due to massive turmoil in the neural and hormonal networks that control the sleep cycle. Dunster et al. tried an experiment where they delayed the start time of the school day from 7:50 to 8:45 a.m. Teenagers got more sleep, school attendance increased, attention in class improved and grades went up 4.5%. This effect is strong, and has been replicated in many places.
Delaying the start time of a school aims to address the biological factors contributing to insufficient sleep and social jetlag, or in other words, the mismatch between adolescent sleep timing and early starts. The movement towards later school start times has received the greatest momentum in the USA, with work done by advocacy groups such as Start School Later and recommendations that middle and high schools should not start earlier than 08.30 [77]. The findings from three articles that have evaluated the body of evidence on delayed school start times are summarized here [78–80]. In a systematic review by Minges & Redeker [78], school start times were delayed by 25–60 min, while weeknight sleep duration correspondingly increased by 25–77 min in the six studies included. Additionally, some studies reported reduced daytime sleepiness, depression, caffeine use and tardiness to class.
That was a lot of information, but I hope this provides a useful collections of resources about learning, and an introduction to key ideas. I believe these four pillars of learning are relatively uncontroversial, and most of the researchers who argue otherwise are usually disputing the quantity of effects, rather than their quality.
The global workspace theory is a theory of consciousness proposed by Bernard Baars, and developed by Stanislas Dehaene and Jean-Pierre Changeux. It’s a macroscopic theory of consciousness, and too complicated to unravel here.